Predicting the Stock Market with Sentiment Analysis
Video Presentation
Table of Contents
- Introduction
- Related Work
- Sentiment-Trading Pipeline
- Results
- Areas of Improvement
- Effort
- Conclusion
- References
- Appendix
Abstract
Predicting the best stocks to buy while day-trading the stock market is a dream for many seeking financial freedom. While there are heaps of existing day-trading strategies, many of them involve long hours of strict human focus on the numerical trends of stock prices and the contextual trends from news sources that directly correlate to the future value of publicly traded companies. We live in an age where there exists a subcategory of machine learning that can help reduce the manual burden of analysis and automate tasks that predict trends quicker than any human. Enter Natural Language Processing (NLP) and the Transformer model for sentiment analysis. This article discusses the use of an NLP pipeline that trains an altered BERT model with tweets from Twitter to make predictions about their bullish and bearish sentiments concerning stocks. The pipeline then conducts trades based on a user’s predefined portfolio and desired risk level.
1 Introduction
The purpose of this project was to create an artificially intelligent stock trading bot that makes decisions regarding the trading of stocks based on the opinions that people share about stocks on Twitter. The word “intelligent” is used to describe how this bot performs because it makes decisions without human intervention using probability. This intelligence is attained using supervised machine learning techniques, which is the process of using pre- labeled data and probability theory to make predictions on unclassified data.
In our case, we have a labeled dataset of Tweets about stocks and their corresponding bearish or bullish sentiment, which was used to train and test the sentiment classification model. The words bearish and bullish refer to the perceived trend of how well the price per share of a publicly traded company is performing on the stock market. Bearish is perceived as poor performance that would decrease the company’s value, and bullish is perceived as strong performance that would increase the company’s value. Trading stocks on these sentiments, before everyone else does, to return a profit would be considered “predicting the stock market.”
1.1 Why Sentiment Analysis?
Many investors are swayed in their trading decisions by news about publicly traded companies, and many of those investors include the analysis of news in their stock trading strategy. Some long-term traders might be more interested in calculating the valuation of a stock based in the company’s performance metrics, while many short-term or day-traders might find including news in their analysis to be beneficial.
1.2 Why Use Twitter as the Source of News?
Often-times a news headline can give us a gist of whether a company is about to make or lose a lot of money, but there are a few reasons why this bot is focused on using tweets from Twitter for sentiment analysis instead of typical well-known news sources, such as The Wall Street Journal.
News articles are long. Predicting the sentiment on a long piece of text can cause a lengthy computation time for our model. This can cause issues for day-trading stocks because time is of the essence. Keeping the analyzed text short and sweet can make for swift analysis and thus quicker trading. Thus, perhaps we could consider just the news headline?
A potentially harmful issue with using just news headlines is “click-bait,” which is a flashy headline that encourages potential readers to click on an article and start reading it. Sometimes the contents of these articles do not align with the potentially misleading headline – which could mean tricking the bot into predicting a bearish sentiment when the article might have bullish sentiment.
Two major downfalls of mainstream news are the low volume of articles that get produced per day and the time it takes for a journalism team to produce it. There are many news sources reporting on the stock market, but their abundance is countably finite and their proofing takes time. Thus, we turn our attention to tweets about stocks on Twitter.
While tweets on Twitter are neither infinite nor factual, the overwhelming volume of opinions that get shared on Twitter every second is appealing due to its fluidity, and people on Twitter tend to express their opinions via tweets about how stocks are performing throughout market hours. This information is valuable because it can be viewed as contextual information for how the market is performing in real time. Natural Language Processing and sentiment analysis can be used to identify trends from these opinions and produce a majority-ruled polarity score for the sentiment of a particular stock. People are relatively quick to post a quick tweet about a major price change of a stock compared to the time it takes for a journalist to post an article about it on the web.
The speed of Twitter relative to typical news stories can be illustrated with an example of a panic-driven sell- off in market shares that occurred on April 23rd, 2013. The sell-off was caused by a tweet from The Associated Press’ Twitter account that read, “Breaking: Two Explosions in the White House and Barak Obama is injured.” At the time, The Associated Press (AP) had about 11 million followers, and the moment the tweet was released, investors had sold stocks in a panic, only to find that the tweet was malicious.
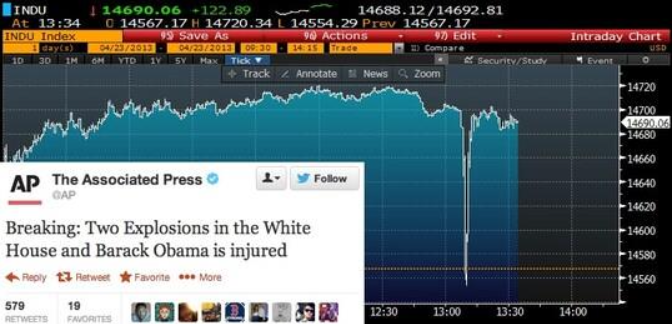
This example plays a major role in why many investors today monitor Twitter for news headlines – because news spreads quickly on Twitter. News stations were not quick enough to cover this 5-minute event, but other market analysts were able to denounce this fake tweet using Twitter much sooner. This example certainly shows a shortcoming of sentiment analysis of tweets. How do we know that a tweet is legitimate? It is also to shows why we are interested in Twitter and not just news headlines – because Twitter news travels faster.
1.3 The Language Model
The sentiment analysis of text builds off the idea of a language model, which is a probability distribution over a sequence of words. Language models have evolved from n-gram models, which struggle with sparse vectors and expensive computation, to neural network models, which struggle contextually with the restrictive window-of-words Markov Assumption, to recurrent neural network models, which struggle with vanishing and exploding gradients, to Long-Short-Term-Memory models, which struggle with lengthy training times due to their recursive and computationally dependent nature, to the rather new Transformer models which reduce training times by allowing for some parallel computation to be done during training.
The BERT model is a popular refined version of the original Transformer model, and because it has gained a lot of popularity for its successes with accuracy and quickness in recent years – it presented itself as an obvious choice for this type of project.
2 Related Work
In 2016, a team of researchers claimed that a strong correlation exists between rise and fall in stock prices of a company and the public opinions or emotions about that company expressed on Twitter through tweets. The main contribution of their work is the development of a sentiment analyzer that can judge the type of sentiment present in the tweet using an n-gram model and a Word2vec model. They concluded their research without any documented application.
In 2017, a team of researchers investigated the simultaneous effect of analyzing different types of news along with historical numeric attributes for understanding stock market behavior. Their model improved its accuracy by considering news with different values of numeric attributes during a day. Three categories of news data were considered: news relevant to market, company news and financial reports that were published by financial experts about stocks. The model consisted of two stages. 1. Determine the news polarities to be either positive or negative using naïve Bayes algorithm. 2. Incorporate the output of the first stage as input along with the processed historical numeric data attributes to predict the future stock trend using K-NN algorithm. Their model achieved an accuracy up to 89.80%. They also concluded their research without documented application.
In 2020, a YouTuber, named “The Code Whisperer” posted a video of himself writing code to scrape tweets from Twitter, analyze their sentiment, and then conduct trades based on that sentiment using the Alpaca trading platform. He appears to classify tweets using a python library called pickle, and he also uses Tweepy for scraping tweets. This video was the primary inspiration for this project.
3 Sentiment-Trading Pipeline
3.1 Tools for Implementation
The entire pipeline is written in Python using multiple libraries. The PyTorch and Pandas libraries are used throughout the program to organize and manipulate tensors and matrices of data used to train the model and make predictions on the sentiment of text. The base BERT Transformer model that is used comes from the developers at hugging face.
The model is initially trained with Google Colab’s GPUs, then its tensors and predictions are saved into files to be used again as a pretrained model that is read into the program. This was helpful to prevent running over Google Colab’s GPU computation limit and allows for the remainder of the pipeline to be quickly run on a local machine’s CPU.
A developer profile on Twitter is necessary to use the pipeline to get access to their API keys, and the Tweepy Python library is used to extract real-time tweets from Twitter’s API.
A stock trading account on Alpaca must also be created to get access to their API keys for trading money on a real or simulated version of the real stock market.
3.2 Data Preprocessing
The first stage of the pipeline is data preprocessing, and the data set used to train and test the sentiment classification model has sample size of 5790 tweets.
The cross-entropy loss function that is used during training requires all target variables to be nonnegative, which required bearish target variables in the dataset to be replaced with a “0” instead of a “-1”.
The data is then split 80-10-10 (train-test-validate) and encoded into vectors before being to be passed into a PyTorch DataLoader for the purpose of properly formatting the data for attention-masking in the BERT Transformer model.
3.3 BERT Sentiment Classifier
The Sentiment Classifier is built on top of the BERT model and includes a dropout layer for regularization. It then returns an output from the last fully connected layer because that is what is required for the cross-entropy loss function used during training. After 10 Epochs of training, the model predicted about 83% of the validation data correctly. So, there’s plenty of room for improvement.
The BERT model computes a likelihood score for both the bullish and bearish classifications for a tweet, and its prediction is simply the larger value from the two. These likelihood scores are represented as floating decimal point numbers and may even be negative to suggest that the classification is very unlikely. For example, the model may assess a tweet with a bearish likelihood score of -0.1 and a bullish likelihood score of 0.1. Thus, the prediction would be bullish, but without much certainty, compared to a bearish likelihood score of -3.0 and a bullish likelihood score of 3.0. This level of certainty will be referenced again later in the pipeline.
Why BERT? The classic LSTM and Transformer models only receive context from the words that have already occurred in the prior sequence of words. The BERT language model is conditioned on the words that occur on both sides of the word to be predicted. This is accomplished without allowing each word to indirectly see itself in a multi-layered context with the use of “masks.” The model masks 15% of the input’s words and has itself predict the missing words using the bidirectional context. Thus, BERT is more successful than other language models with identifying the sentimental differences between two sentences with similar words, yet different meaning, such as: “work to live” vs. “live to work.”
3.4 Live Tweet Preprocessor
Queries are made for tweets from Twitter’s API using the Tweepy Python library. The pipeline arranges a collection of stock ticker symbols into a Python dictionary as keys for the purpose of querying them to find tweets that contain those stock symbols.
A forever running while-loop cycles through querying each of the stock symbols one at a time, and the Tweepy module initially returns a JSON formatted data frame of fixed length from the API. The data frame consists of the tweet text, a timestamp, a tweet id, the language, and much more. The tweet id is an integer associated with uniquely identifying each tweet that has ever been posted on Twitter, and as you might imagine, this number has gotten very large, as it increments by one with the creation of each new tweet. With each call to the API, the largest id is stored as a value in the stock symbol dictionary associated with the stock symbol key. Now, each subsequent query to the API makes sure to include that only tweets with an id greater than the one stored in the dictionary should be returned, thus avoiding the future analysis of duplicate tweets.
From here, each tweet text from the JSON structure is “cleaned” using regular expressions, and then organized into a Pandas data frame, keeping only the tweet text and the tweet id.
An area that requires more investigation is the topic of retweets. A retweet is an unoriginal post on Twitter that people use to re-post a message that was shared by the original tweeter. The twitter query returns these retweets as duplicate instances of text with a “RT” prefix. These may or may not be something to remove from the analysis. For this pipeline, the retweets were kept with the thought that it may be important to capture the essence of popular tweets that get repeated multiple times, which emphasizes their importance on a stock’s sentiment. Retweeting can be a sign of agreeing with the sentiment from another user, and that sentiment can have more weight on deciding whether to trade a stock if it gets repeated across Twitter. So, for now, retweets are considered valuable information not to be filtered.
3.5 Live Tweet Sentiment Prediction
The method that handles prediction of tweet sentiment accepts as arguments the sentiment classification model, the Pandas data frame of recent tweets, and the user’s desired risk level for stock trading, which is a value between zero (most conservative) and one (most risky).
Next, it determines whether to trade a stock based on the certainty of the model’s prediction about the sentiment of tweets it has just analyzed. If the user sets their desired risk level closer to zero, then the BERT model is required to be more certain about its prediction before making a trade, thus decreasing the volume of trades that occur. Since the BERT model computes a likelihood score for both the bullish and bearish classifications for a tweet, certainty is defined as the difference between the two scores.
The method also keeps a tally for a total polarity score for the incoming data frame, which increments by one with each certain bullish prediction and decrements by one with each certain bearish prediction. Bullish and bearish predictions that do not meet the minimum level of certainty specified by the risk level do not affect the polarity score.
The absolute value of the polarity score functions as a multiplier that contributes towards the decision of how many shares to buy of the specified stock. Thus, a polarity score of zero multiplies by zero, which results in not making any trades with that stock. Essentially, a score of zero means that the accumulated sentimental predictions of certainty from all of the tweets in the data frame was neutral, and this begins to happen more often as the user decreases the input of the risk level of their trading strategy.
3.6 Live Stock Trader
The method responsible for making trades communicates with the Alpaca API. Initially, the trading bot checks if the market is even open for making trades so not to place orders that would otherwise stay pending outside of market hours until the market opens again, when the sentiment has likely changed.
Next, if the predetermined polarity score is not zero, then the bot will start determining how many shares to trade and what type of trade to make. The number of shares to trade is a function of the polarity score, the price of the stock being traded, the account remaining buying power, and the accounts current value or equity.
Before buying shares of a stock, the bot notes the current approximate price of the stock, then calculates a value equivalent to 1% of the current portfolio value divided by the current price of the stock, which is then multiplied by the polarity score to determine the quantity of shares to buy. This expense of this quantity of shares is then compared to the account’s current available buying power. If the buying power is exceeded by this amount, then the quantity is decremented by one share until the expense is affordable for the account to make the purchase or the quantity of shares to buy is zero.
Before selling shares of a stock, the bot needs to determine if it even owns any of that stock to avoid throwing an error by trying to sell something it does not own. If it does own that stock, it then decides to sell the quantity of shares in the same way that it determines the number of shares to buy, using a combination of polarity score, portfolio value, and current approximate price per share. If that quantity is greater than the number of shares currently owned, then the bot simply sells all of that stock.
With all this math and fact checking, there is still room for error because the current stock price is always an approximation since traders are buying and selling stock at various prices within milliseconds of each other. Thus, the order is placed within a try-except block and marked as a valid trade once complete, inspired by how mutual exclusion locks work with parallel programming systems. If an error is thrown because the trade expense is suddenly too expensive within milliseconds, the bot decrements the quantity of shares to buy by one and tries again. If the quantity decreases to zero before it becomes affordable, then the transaction is marked as “skipped”, terminates the trading process, and exits the function.
Along with a time stamp, the function returns one of the following messages at the end of its run:
- Success! Order placed to {buy/sell} {quantity} shares of {ticker}.
- Trade failed. Alpaca account status: {open/suspended}.
- Transaction prices changed during processing. Either not enough buying power or insufficient shares to sell. Skipping.
- You don't have enough buying power to buy {ticker}. Skipping.
- You do not own any shares of {ticker} to sell. Skipping.
- No orders were made because the stock market is currently closed for trading.
4 Results
The first day of trading, which occurred on Monday, August 25th, 2021, was a success up 1.8% using a predefined risk level of 0.2. It was generally a good day for most people trading that day. The S&P500 and DJIA were up 0.85% and 0.61%, respectively. The bot did produce higher returns than the S&P500 and the DIJA, although this is largely due to the stocks that were picked simply doing well that day.
The following table displays the stocks in the bot’s portfolio, and their price changes for that day. Note that these were the only stocks that the bot was allowed to consider as predefined by the user. Also note once more for clarity that the following table is not describing the bot’s portfolio, but rather each stock’s price change for the day.
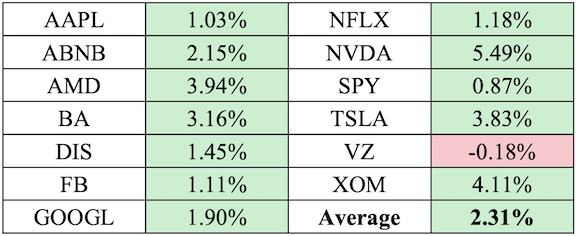
One indication of negative performance in my bot includes the observation that if equal amounts of money were spent buying each of the stocks in the portfolio at the beginning of the day and held onto until the end of the day, then the “uniform-portfolio” return would have been about 2.3%, which is a lot higher than the 1.8% that the bot returned.
However, there is also a clear sign of positive performance. The key idea is to notice the correlation between the market value of stocks in the portfolio and the sentiment of stocks that did well that day, and what is meant by that is the sentiment of tweets from Twitter guided the trading bot in the right direction to buy more stocks with more bullish sentiment, and shy away from stocks receiving a lot of negative sentiment.
To Illustrate this key idea, we’ll look at three of the top four performing stocks in the portfolio from that day, namely NVDA, AMD, and TSLA. The “market value” for a stock in a portfolio is the number of shares owned times the market price for that stock. Thus, we hope that the bot has placed most of its money into stocks that it has seen receive the most bullish sentiment on Twitter. Since our bot is using sentiment analysis - It is no coincidence that the bot learned from Twitter to buy more shares of NVDA (+5.94%), AMD (+3.94%), and TSLA (+3.83%), which are the stocks with the highest market value in the portfolio.
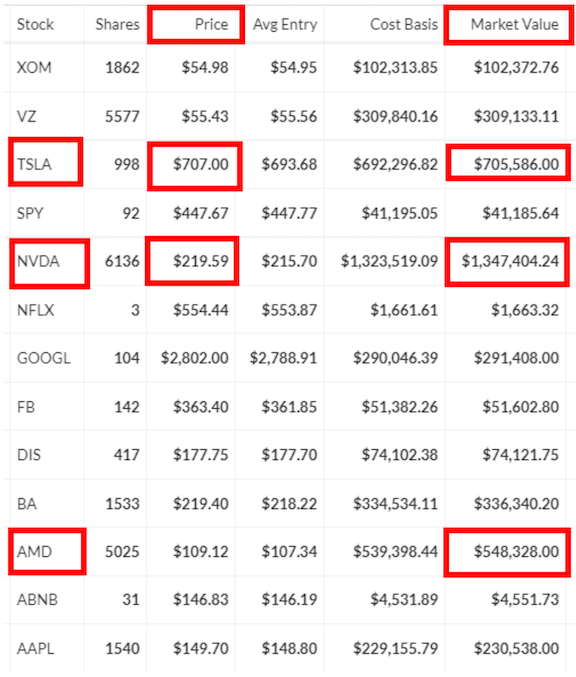
Given the portfolio shows the market value for these three stocks is higher than all the other stocks in the portfolio. we can infer that the bot intelligently learned from tweets that these three stocks were looking bullish that day, and it acted accordingly.
Now, one might assume that the market value for a stock in the portfolio is correlated with the price per share of that stock such that a more expensive stock, such as TSLA, would act as a larger multiplier towards more money invested in that stock, but as mentioned earlier, the bot’s stock purchasing function is programmed to scale down the number of shares to buy when a stock is more expensive. This is illustrated by noticing how shares of NVDA cost less than shares of TSLA, so the bot scaled up the number of shares it could afford per bullish polarity point when buying NVDA.
To summarize this big idea - the bot’s intelligence is coming purely from the sentiment of tweets because it has absolutely no idea how well a stock is performing when it decides to trade it. It doesn’t get any input about its trending price or any other kind of financial data; it only makes decisions based on the live opinions from people on Twitter.
Of course, this bot is clearly not perfect because it missed a beat with the Exxon Mobile stock (XOM), which was up 4% that day.
5 Areas of Improvement
The prediction accuracy would benefit tremendously from a larger data set than 5,790 tweets. It is a good start, but it is a relatively small sample size, and may be causing some overfitting. Additionally, the implementation of cross validation splitting on the data could do no harm.
Through patient trial and error and perhaps multiple GPU’s, one could find a more optimal combination of adjustable model parameters, such as learning rate and the dropout layer.
There is a surprisingly large number of variables that go into a search query for tweets from the Twitter API. This pipeline simply queried for ticker hashtags one at a time, but often -times twitter users included multiple ticker tags within the same tweet, which may or may not be throwing off the prediction. This can be avoided by adjusting the query function to filter out stock symbols in tweets for a specified symbol query. Figuring out a good way to handle this issue would require some experimentation.
There’s a lot of improvement to be made with the actual stock trading strategy. For the sake of making this more of an NLP project instead of a stock trading project – there really wasn’t any special strategy. The bot uses simple market orders on a portfolio of stocks that have very little diversity. A handful of well-known stocks were chosen, and it just so happens that most of them are tech companies. So, there’s a lot of strategy left to play with, such as the use of limit ordering, and fine tuning the bot’s way of determining how certain it is with its predictions, which directly affects how many shares of a stock to trade.
Additionally, the bot purposely pauses between queries so not to exceed query limits on Twitter and Alpaca, so there is about 9 seconds between each stock analysis. Thus, a portfolio of 13 stocks takes about 2 minutes to cycle through, which creates about a 2-minute window for disaster to strike a particular stock before the bot notices. So fine tuning the wait times to maximize queries without exceeding limits is critical for users with larger portfolios.
A farther-reaching goal would be to have sentiment analysis play a complementary role in an ensemble of machine learning models to make good trading decisions, such as weighing sentimental predictions against a stock’s historical price trend.
6 Effort
The decision on which language model to use transitioned almost synchronously with the completion of each Natural Language Processing homework assignment at The University of Chicago. Initially the use of a CNN was of primary interest since the details of its functionality were clear from a previous machine learning class. This desire quickly switched to using an RNN due to a bias towards it after spending considerable time learning about it. Finally, one last transition was made to using a Transformer after studying it more recently and considering its resume appeal for being a relatively hot topic in the field.
Additional understanding was necessary to use the BERT Transformer, which was accomplished through reading 𝑇h𝑒 𝐼𝑙𝑙𝑢𝑠𝑡𝑟𝑎𝑡𝑒𝑑 𝐵𝐸𝑅𝑇 and Hugging Face’s documentation for BERT using PyTorch.
The Tweepy library, Twitter API, Alpaca API, are all new skills. Tweepy’s documentation is well-written, so it was not difficult to find what was needed to complete that section of the pipeline. Getting approved for a developer account through Twitter was much more of a hassle. An initial application was required, as well as multiple emails back and forth with the Twitter team to specifically verify the intentions for using their data. The documentation for how to use the Alpaca API is incomplete or lacking. There is however a community forum and Slack page, where undocumented code was discovered thanks to other developers who were so gracious to answer questions.
Time spent on this project initially started with the reading of research papers and watching YouTube videos to get an idea for what could be possible. Once the pipeline started coming to fruition, little time was spent reading other work compared to the fine tuning of this pipeline’s parameters and reading documentation on the dependencies for this project.
7 Conclusion
After three days, the bot’s portfolio value has increased from $1M using $4M in buying power to $1.08M, but it will take many more days of evaluating the bot’s performance to determine if it is a good stand-alone stock trading model that can be relied upon to multiply one’s investments without intervention.
There are plenty of simple improvements to be made before considering the tremendous risk of using real money.
References
- Ashish Vaswani, Noam Shazeer, Niki Parmar, Jakob Uszkoreit, Llion Jones, Aidan N Gomez, Lukasz Kaiser, and Illia Polosukhin. 2017. “Attention is all you need.”
- Jacob Devlin, Ming-Wei Chang, Kenton Lee, and Kristina Toutanova. 2019. "BERT: Pre-training of Deep Bidirectional Transformers for Language Understanding".
- Max Fisher. 2013. “Syrian hackers claim AP hack that tipped stock market by $136 billion. Is it terrorism?”
- Venkata Sasank Pagolu, Kamal Nayan Reddy Challa, Ganapati Panda, Babita Majhi. 2016. "Sentiment Analysis of Twitter Data for Predicting Stock Market Movements".
- Ayman E. Khedr, S.E.Salama, and Nagwa Yaseen. 2017. "Predicting Stock Market Behavior using Data Mining Technique and News Sentiment Analysis"
- The Code Whisperer. 2020. "I Coded A Twitter Sentiment Analysis Trading Bot And Let It Trade!"
- Hugging Face – BERT Transformer Library
- Twitter Developer Platform - API documentation.
- Tweepy Python library - API documentation.
- Alpaca trading platform – API documentation.
- Jay Alammar. 2018. "The Illustrated BERT, ELMo, and co. (How NLP Cracked Transfer Learning)".
- Khan Saad Bin Hasan. 2019. “Stock Prediction Using Twitter”.
- Qiurui Chen. 2020. “Language Models and RNN”.
Appendix
A.1 Benchmark Results
The source code for this project with instructions on how to run the web application can be found in my GitHub repository.
A.2 Video Presentation
The video presentation for this article can be found here on YouTube.